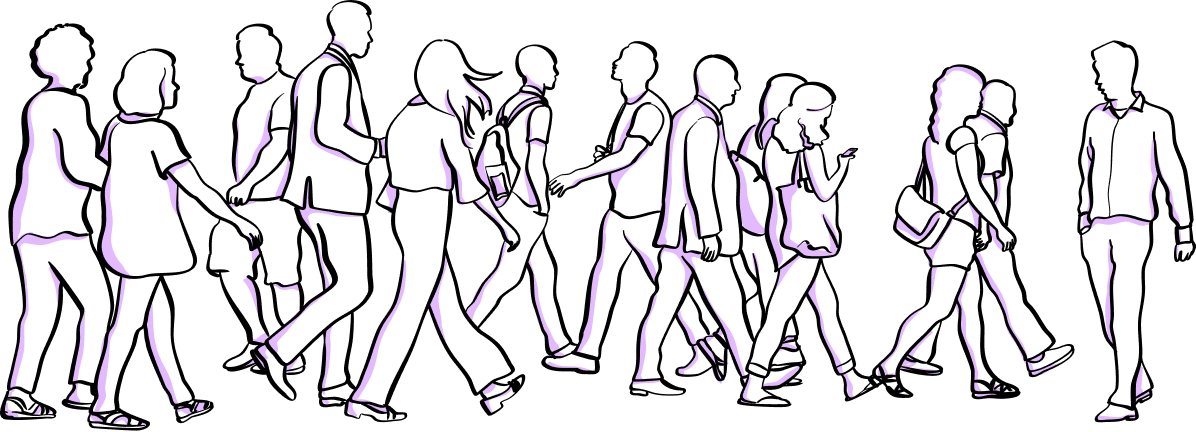
Unified data and AI for predicting customer behavior
Score leads, recommend next actions, forecast spend, detect churn, and more.
Built-in consumer data
Gain a panaromic understanding of your customers with 1,500+ consumer attributes on nearly 270 million adults.
Intuitive prediction
Choose the customer behavior you want to predict. The platform will determine the best method, from GBT to GenAI.
AI safety
Built-in bias mitigation, explainable AI, and more.
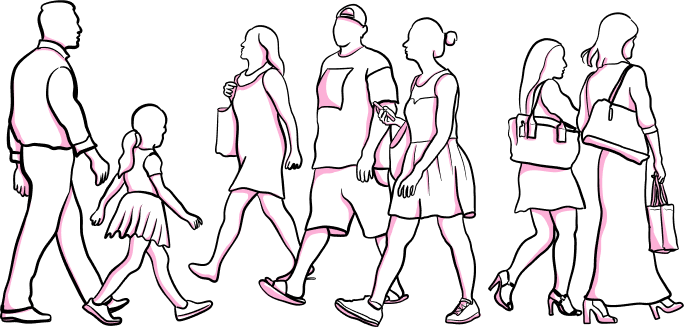
Stack integrations
Faraday integrates with the rest of your stack to ensure your predictions are being incorporated where and when they’re needed.
NEW Powered by Faraday — for software teams
Quickly ship the predictive features your users crave
Developer ready
Powerful, ergonomic API for easily predicting customer behavior. Build and ship fast.
Identity resolution
Combine data from your sources for the most accurate predictions possible.
Deployment options
Use our secure hosted option (deploys daily!), or launch on your private cloud.
Security & privacy
SOC-2, NIST 800-53, CCPA, HIPAA, and more to keep your data safe.
Prediction recipes
Try predictions in your workflows with our Zapier plugin